Data-driven organizations are 23x more likely to acquire customers, six times as likely to retain customers, and 19x more likely to be profitable.
This makes a strong case for implementing business analytics in your org. This article discusses how you can use advanced analytics and AI to grow your business’ revenue.
We cover:
- Why business analytics are important in RevOps
- The four pillars of business analytics
- Bringing them together
- Using AI in business analytics
Why are business analytics important in RevOps
Utilizing business analytics within your revenue operations strategy can allow you to make better-informed decisions, improve data hygiene, and precisely forecast revenue.
The four pillars of business analytics form a data maturity model that allows your organization to increase its data capabilities over time. This serves as the foundation of data-driven decisions.
The four pillars of business analytics are:
- Descriptive
- Diagnostic
- Predictive
- Prescriptive
As your organization moves through this model, your data complexity increases and data hygiene becomes more important for accurate results.
Implementing business analytics within your revenue function ultimately leads to a mature data organization with advanced data-driven decisions and accelerated revenue growth.
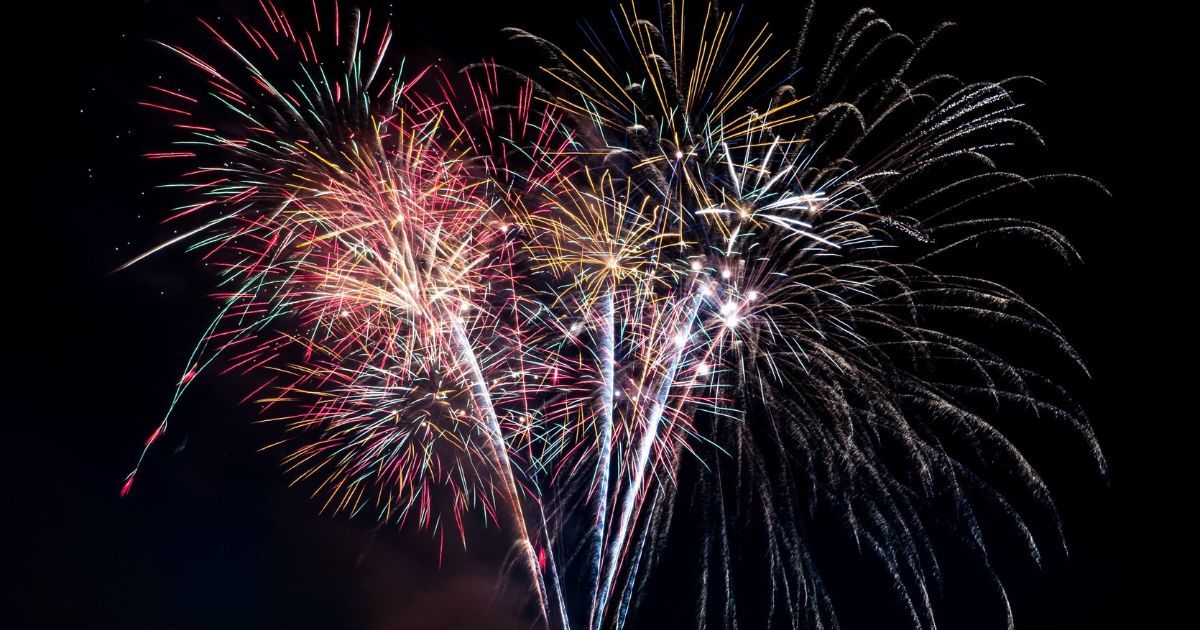
Descriptive analytics
Descriptive analytics are observations of data that describe what happened in your organization. This is the simplest type of analytics, but it's a vital starting point when ramping up your business analytics maturity model.
Essentially, descriptive analytics are the raw metrics that describe how your team performed, such as their KPIs.
How to use descriptive analytics
Descriptive analytics are essential to track performance month-on-month and show improvements in your revenue strategy.
These are often the metrics shown on dashboards teams use for monthly reporting, for example, annual recurring revenue, win/loss rate, email open rate, churn rate, website traffic, and so on.
To get descriptive analytics right it’s important to be competent in data modeling, communicating data with the right visualizations, and basic dashboard design.
Use cases
As we’ve mentioned, the main use case for descriptive analytics is monthly KPI reporting across revenue-generating teams in your business.
For example, creating a sales report for the sales leadership team to track your company’s revenue growth.
While this is an important use case, it’s important to look beyond these basic numbers and dive into the why.
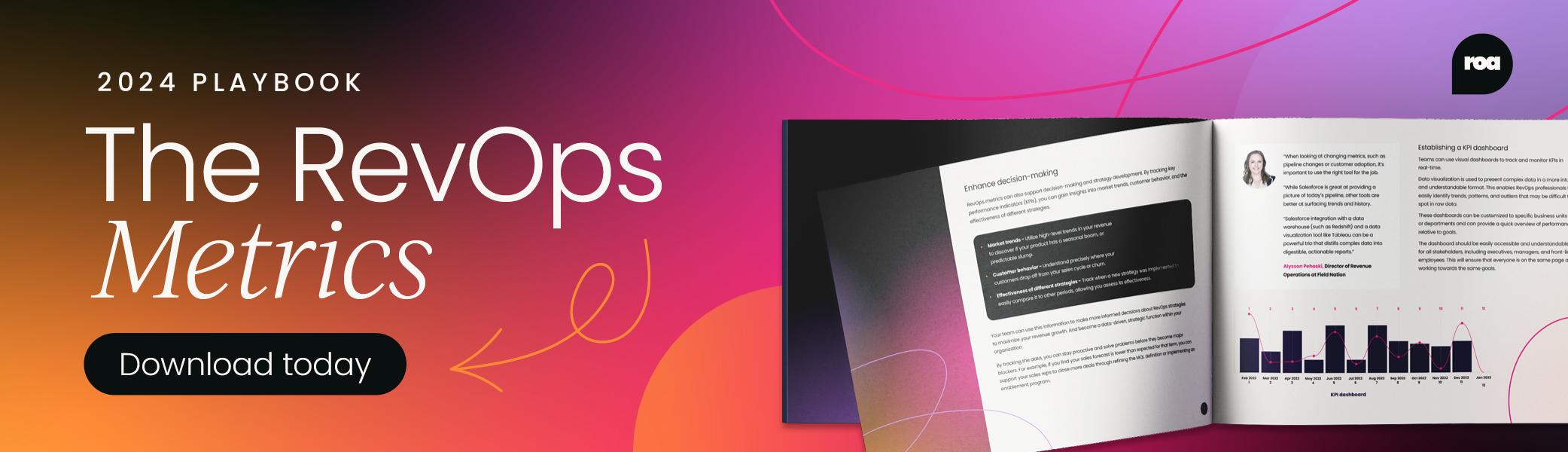
Diagnostic analytics
Enter diagnostic analytics. 😎
Diagnostic analytics uncover why an outcome occurred, and what is likely to have caused the change. Whether you’re diagnosing a win or a loss within a key metric, this type of analysis can pinpoint the cause.
This takes descriptive analytics to the next level by pinpointing the cause of your data to shift.
How to use diagnostic analytics
Diagnostic analytics often make use of software to easily pull out these insights from your company’s data.
This process typically involves leveraging data drilling, data mining, and correlation analysis, which can be complex without help from an analytics tool.
Use cases
A common use case in revenue operations is trying to understand an unexpected rise in churn rates. Diagnostic analysis can help you uncover the changes in customer behavior, customer service response times, and other factors that might’ve caused your customers to churn.
Diagnostic analysis can also help your team to reflect on wins. If sales reps drastically increase their deals won, you can analyze their length of calls, number of emails, time for contract approval, and more to assess what worked well.
Plus, if your company uses conversation intelligence tools, diagnostic analysis can also consider what your seller said to clients.
This can help you identify your organization's best sales strategy and inform the sales enablement strategy.
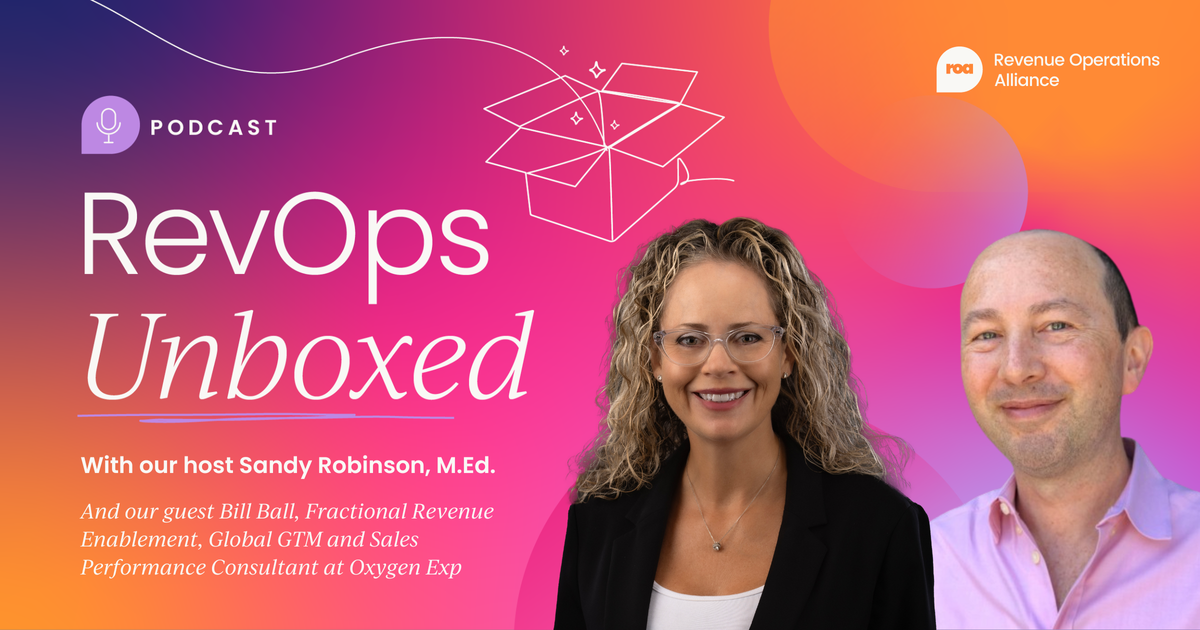
Predictive analytics
Predictive analytics asks what’s likely to happen based on historical data. It can help you predict outcomes and test theories using machine learning and AI. These predictions can also help you spot emerging market trends in your industry.
While data hygiene is important for all types of analytics, the errors of poor data are compounded in predictive analytics, so it’s important to use accurate data to produce as accurate a prediction as possible.
How to use predictive analytics
There are a few steps involved in predictive analytics:
- Identify the problem and define what to predict.
- Collect the data you’ll need for analysis.
- Organize and cleanse the dataset.
- Define your objective (what will you achieve through prediction?).
Use cases
The most obvious use case for predictive analytics is in sales forecasting. By compiling sales pipeline data, AI can accurately predict what your revenue will look like next quarter.
This can allow data-backed decisions around sales strategy, budgets, and other key business decisions.
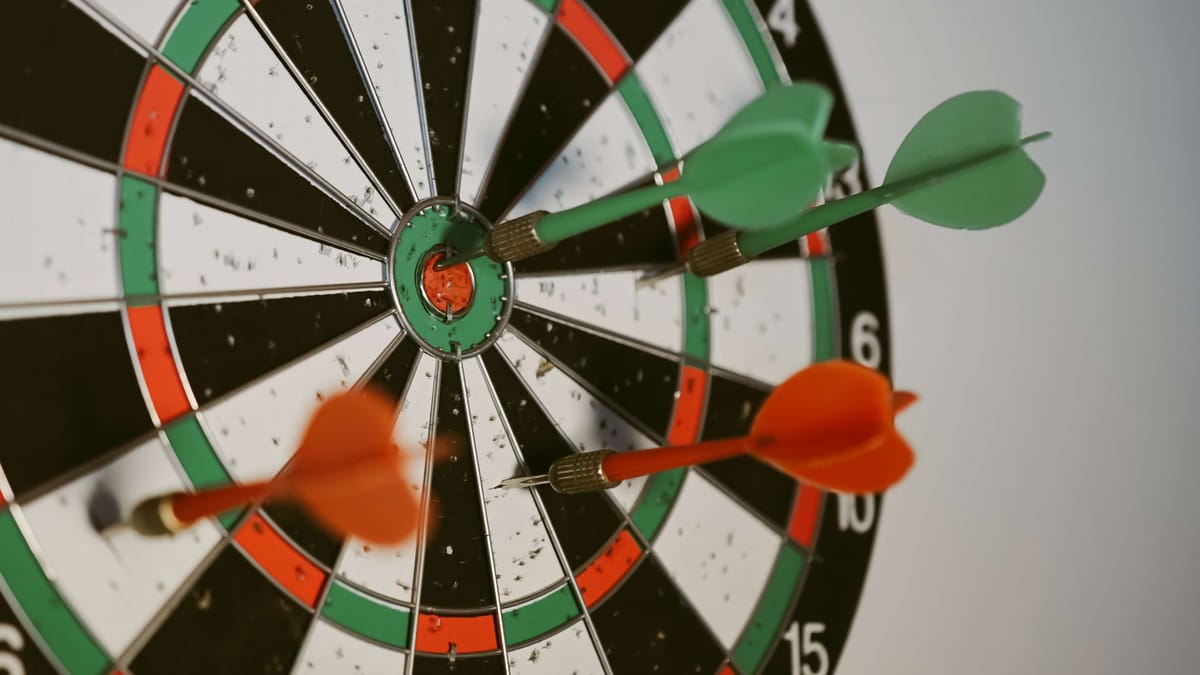
Prescriptive analytics
Prescriptive analytics is the most powerful form of business analytics, as it allows the data to tell you the best course of action for your business.
This data takes into account the insights from the other stages and compiles them with AI to suggest the best corrective action to get to your desired business outcome, such as reduced churn rates, or increased win rates.
A basic example of prescriptive analytics you’re likely familiar with is Google Maps’ directions feature, as it outlines the best way to get from A to B within your preferences (eg. walking, public transport, avoiding highways, etc.).
With this concept applied in your organization, you can make true data-backed decisions.
How to use prescriptive analytics
Prescriptive analytics isn’t something you can jump straight into, it’s crucial to build up your organization’s data maturity first. Without a strong data foundation for your recommendations, they’re likely to be vague, or unhelpful.
Increasing your accurate data points will drastically improve the quality of your AI tool's recommendations.
Use cases
If your organization’s product fluctuates in demand or is highly seasonal, you could use prescriptive analytics to adjust your pricing to account for these changes.
Some real-life examples of this include Uber and Ticketmaster’s use of dynamic pricing. But fair warning, as both of these dynamic pricing strategies have proven controversial in the past, it’s still important to run these changes past a human for final sign-off.
Another use case of prescriptive analysis is flagging employees who may need some additional training or support to your sales enablement team.
If your AI tool spots a trend in your sales rep’s performance it could automatically enroll them in a specific enablement program to improve their performance.
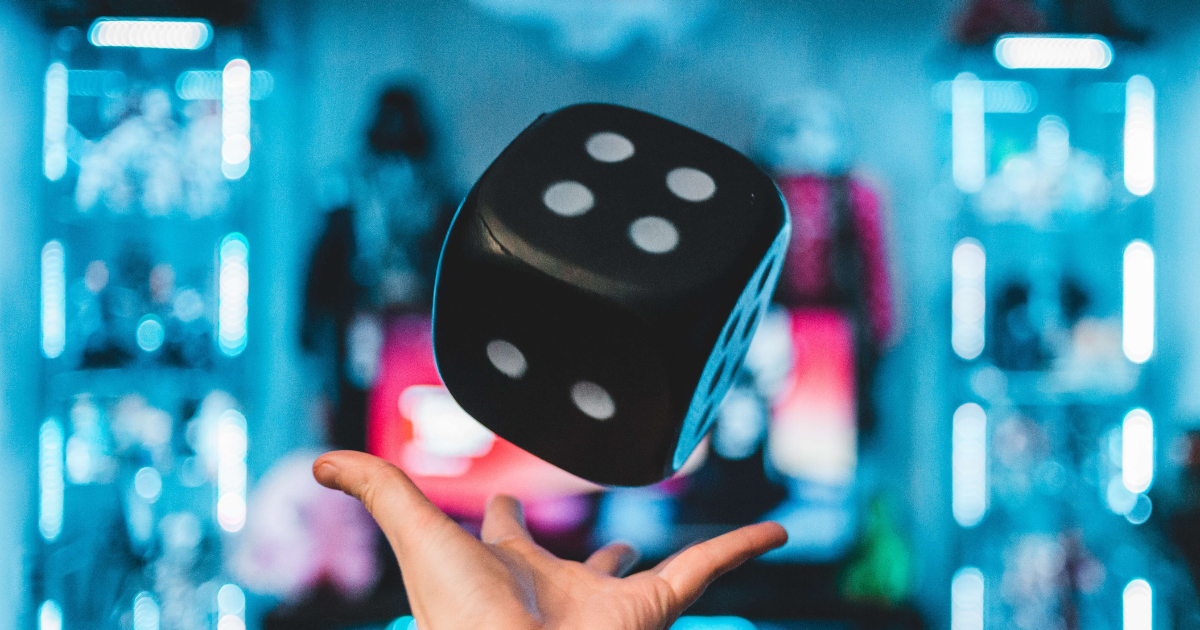
Bringing them together
We’ve hinted at this already but these four pillars come together to create an unstoppable data-powered organization.
By working your way along these four pillars of the data maternity model, your organization will unlock new data capabilities to ensure your revenue team can make informed decisions.
From sales and marketing strategy to training your team, going beyond descriptive analysis allows you to solve problems, predict future outcomes, and discover the best course of action (according to the data).
So long guessing game, hello data-informed decisions! 😍
Improving data analytics with AI
To make the most of your data, consider onboarding an AI tool to support your analysis. AI can provide insights quickly, and prevent human error from skewing your results.
Revenue intelligence software is a game changer when analyzing your revenue data, quickly pulling out actionable insights from a ton of data. But make sure to select the tool that’s best suited to your business needs and budget requirements.